The current capabilities of Artificial Intelligence (AI) have expanded beyond computing. AI has branched out to assist in making modern tasks faster and easier, even in agriculture. Today, AI plays a key role in improving agricultural productivity, offering valuable support to farmers, such as in the classification of mangosteens.
The “Automatic Classification of Mangosteens and Ripe Status in Images Using Deep Learning Based Approaches” program is part of the project “Automatic Grade Assessment and Translucent Flesh Disorder Detection of Mangosteens from Capturing Images using Deep Learning and Image Processing-based Approaches,” which includes another program developed alongside it—”Automatic Grade Assessment and Translucent Flesh Disorder Detection of Mangosteens.”
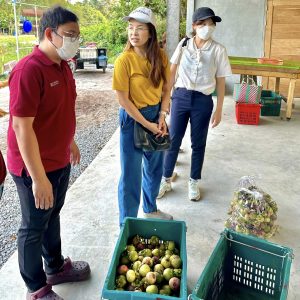
Both programs are in collaboration between the Faculty of Information and Communication Technology at Mahidol University (ICT Mahidol), led by Assoc. Prof. Dr. Worapan Kusakunniran, the Head of the Machine Vision and Information Transfer Lab (MVIT) and Program Chair of the Ph.D. in Computer Science, and the School of Agricultural Resources at Chulalongkorn University. The project originated from a desire to integrate information technology with agricultural management. This study focuses on the classification of the quality of mangosteens, a major economic crop of Thailand that is widely exported.
“There are various methods for classifying mangosteens. The common method involves manual sorting, where a large batch of 100-200 mangosteens is poured onto a large tray, and people sort them one by one. However, each person has a different standard for sorting due to their individual learning processes. This results in different grades of mangosteens and affects the time required. In certain seasons, the sorting must be done overnight because if the mangosteens are stored for half a day, they will change color and ripen. If farmers want to sell at a higher price, they need to sell them while they are still green, as this indicates they are not fully ripe.”
While there are machines available for sorting mangosteens, these machines can only sort by size. They lack the ability to classify by grade, color, and the fruit’s internal quality. The larger the mangosteen, the more detailed the sorting required. What will aid in further sorting the quality of mangosteens is the use of a camera-based sorting system.
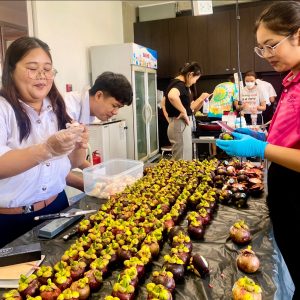
The research team has developed a camera system using Machine Learning and Image Processing. They began by inputting images of mangosteens and designing a Convolutional Neural Network (CNN) architecture based on the knowledge of experts and farmers. This was then adapted into a Machine Learning Model that can predict the grade of mangosteens and assess the likelihood of translucent flesh, resinous flesh, or rot, based on external characteristics.
During the study, Assoc. Prof. Dr. Worapan explained two key challenges encountered during the research: the project’s timeline, as mangosteens are seasonal and not available year-round, and variations in grade classification, as mangosteens differ from region to region. For example, in the studied area, all the mangosteens were of high quality, so there were no samples of resinous or rotten flesh to study. However, this diversity highlighted the differences in mangosteens across Thailand.
Lastly, Assoc. Prof. Dr. Worapan discussed the future of AI in agriculture: “In applying AI, whether in medicine, sports, agriculture, or livestock, we need to understand what we want AI to do in place of humans. In agriculture, our research team aims to make many processes in farming fully automated with AI, to reduce waste, increase operational standards, and raise the quality of agricultural products from Thailand. The goal is to develop devices that help ensure agricultural products meet export standards, improve labor skills, increase product quality, and elevate the image of Thai agriculture to match international standards, like Japan or Europe.”
The “Automatic Classification of Mangosteens and Ripe Status in Images using Deep Learning-based Approaches, Multimedia Tools and Applications (MTAP)” and the “Automatic Grade Assessment and Translucent Flesh Disorder Detection of Mangosteens from Capturing Images using Deep Learning and Image Processing-based Approaches” were both copyrighted in February 2024 under registration numbers W1.011025 and W1.010995.
The research team comprises Assoc. Prof. Dr. Worapan Kusakunniran, the Head of the MVIT Lab, ICT Mahidol; Asst. Prof. Dr. Pimpinan Somsong from the School of Agricultural Resources, Chulalongkorn University; Mr. Kittikhun Thongkanchorn Computer Technical Officer (Senior Professional Level) from the Technology Infrastructure Department (ICT Alumnus #1); Mr. Thanandon Imaromkul (ICT Alumnus #14); and Mr. Kittinun Aukkapinyo (ICT Alumnus #14).
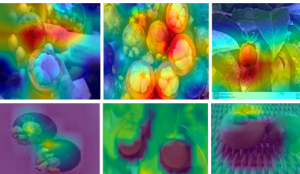
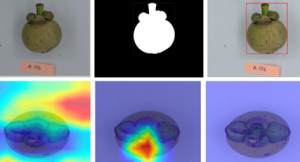
The published research “Automatic Classification of Mangosteens and Ripe Status in Images using Deep Learning-based Approaches, Multimedia Tools and Applications (MTAP)” can be downloaded at >>> https://doi.org/10.1007/s11042-023-17505-0
MVIT Lab’s research can be followed at >>> https://sites.google.com/mahidol.edu/mvit-ict-mahidol“